ERC Consolidator Grant High Dimensional Inference for Panel and Network Data
- ERC Consolidator Grant 2018
- Acronym: PANEDA
- Number: 819086
- Title: High Dimensional Inference for Panel and Network Data
- Initial Host Institution (01/08/2019 - 31/12/2020): University College London (UCL)
- New Host Institution (01/01/2021 - end of project): Oxford University (with UCL still a beneficiary)
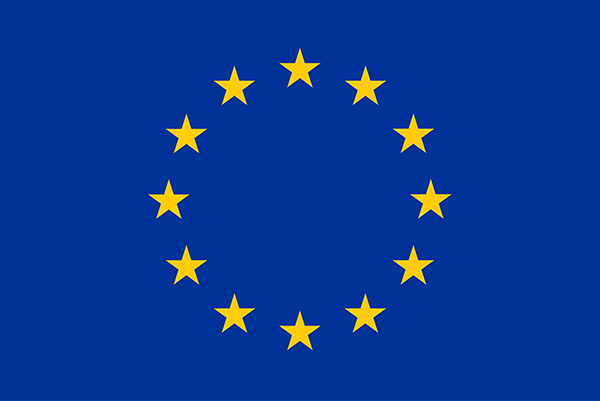
Team
- Principal Investigator: Martin Weidner
- PhD student: Hugo Freeman (on a 48 months PhD stipend)
- Future Postdocs (01/08/2022 - 31/07/2024): Ayden Higgins, and Ben Deaner
- Occasional RA work: Zhuhan Jin (MPhil student in Oxford), Riccardo DAdamo (PhD student at UCL)
Motivation and Goal
The classic data structures that are discussed extensively in the Econometrics literature are either cross-sectional, time-series, or panel data (also called longitudinal data). The basic statistical methods that we use to analyze those data structures have been developed in the 20th century, driven by the availability of corresponding datasets. For example, the systematic collection of stock market data started in the early 20th century, accurate national accounts became increasingly available since the 1930s, microeconomic survey data have been collected systematically since the 1940s, and large longitudinal surveys of households were started in the 1960s.
The trend to increased data availability in the Social Sciences has accelerated in the past decades: Better computer and storage capabilities allow to record and manage much larger datasets, and to access them more easily. We all create digital data footprints on a daily basis, from bank transactions to social network data. New machine learning methods allow to quantify everything from satellite images to legal documents, thus creating structured information out of unstructured raw data. And of course, there have been many conscious efforts of scientists and policy makers to collect larger and better datasets.
Those modern datasets often have a more complicated internal structure that cannot be accurately classified simply as cross-sectional data, time-series data, or panel data. Instead, the structure of the data and of the models that we use to analyze them increasingly have the following characteristics:
- Multiple populations: We observe samples from multiple populations of economic units (eg households, individuals, firms, products, markets), instead of just from one population.
- Network structure: An observation in the data corresponds to a match of multiple economic units, and we observe data for some matches but not for others. For example, we observe wage data for worker i in firm j only for a small subset of all possible matches (ij). The matches that are realized define a network.
- Large number of parameters: The total number of observations is large, but the total number of parameters in the model we want to estimate is also large, because we want to control for unobserved heterogeneity in the form of fixed effects (or other heterogeneous parameters), or because we use semi- or nonparametric methods to avoid strong parametric model assumptions, or have many covariates.
- Parameter specific effective sample size: Only a small subset of all parameters enter into the model for any particular observation. This also implies that the effective sample size for any given parameter is typically much smaller than the total number of observations. For example, a firms fixed effect only affects the observation for that specific firm, and only those observations are helpful to estimate that fixed effect.
- Parameter-data network: We can think of the model parameters themselves as forming a network, where two parameters are linked if there is an observation that is affected by both parameters. If the parameters are fixed effects, then this more abstract parameter-data network simply coincides with the more tangible network generated by the matching process. But the parameter-data network matters for statistical inference more generally, because the noise in the measurement of one parameter affects the precision with which we can estimate any other parameter that is linked to it.
The main goal of this research project is to develop robust inference methods for such sparse panel and network datasets described above. This requires to establish a mathematical representation of the network that allows to formalize asymptotic inference results for sequences of growing networks. In addition, new bias correction and robust standard error estimation methods will be developed that account for the sparsity structure of the data. We will also advance more parsimonious modeling and estimation approaches for situations where the data are otherwise uninformative for the parameters of interest.
A first step towards understanding the connection between the network structure of the underlying dataset with the precision of statistical inference was made in this paper: Fixed-effect regressions on network data. This project aims to extend those results to more general data structures and models. For some models, this means to first establish more credible inference and improved bias correction results for classical (non-sparse) panel and network datasets, but ultimately our goal is to tackle sparse panel and network data.
Publications
- Nonlinear Factor Models for Network and Panel Data, Mingli Chen, Ivan Fernandez-Val, Martin Weidner, Journal of Econometrics, Volume 220, Issue 2, February 2021, Pages 296-324, open access through publisher
- Low-Rank Approximations of Nonseparable Panel Models, Ivan Fernandez-Val, Hugo Freeman, Martin Weidner, Econometrics Journal, Volume 24, Issue 2, May 2021, Pages C40-C77, open access through publisher
- Bias and Consistency in Three-way Gravity Models, Martin Weidner, Thomas Zylkin, Journal of International Economics, Volume 132, September 2021, 103513, open access through publisher
- Network and Panel Quantile Effects Via Distribution Regression, Victor Chernozhukov, Ivan Fernandez-Val, Martin Weidner, Journal of Econometrics (published online), open access through publisher
- Posterior Average Effects, Stephane Bonhomme, Martin Weidner, Journal of Business & Economic Statistics (published online), open access through publisher
Working Papers
- Minimizing Sensitivity to Model Misspecification, Stephane Bonhomme, Martin Weidner, accepted at Quantitative Economics, arXiv version
- Moment Conditions for Dynamic Panel Logit Models with Fixed Effects, Bo Honore, Martin Weidner, arXiv version
- Dynamic Ordered Panel Logit Models, Bo Honore, Chris Muris, Martin Weidner, arXiv version
- Linear Panel Regressions with Two-Way Unobserved Heterogeneity, Hugo Freeman, Martin Weidner, arXiv version
Statistical software packages based on the above publications and working papers
- A stata package based on the paper Bias and Consistency in Three-way Gravity Models was written by Thomas Zylkin and is availble here: ppml_fe_bias
- Software packages for some of the other publications and working papers above are in preparation.
Selected Presentations
- Nov 2020: Keynote presentation by PI at the conference Modelling with Big Data and Machine Learning: Measuring Economic Instability, Bank of England (virtual meeting).
- July 2021: Keynote presentation by PI at the 26th International Panel Data Conference (virtual meeting).
Conferences
- The workshops planned for 2020 and 2021 had to be canceled due to the covid-19 pandemic.
- 2022 Panel Data Workshop: June 6 & 7, 2022, in Oxford. Presenters: Thierry Magnac, Eleni Aristodemou, Bo Honore, Cavit Pakel, Jiaying Gu, Victor Aguirregabiria, Bryan Graham, Chris Muris, and Koen Jochmans. Website.
- 2023 Workshop on Applications of Random Matrices in Economics and Statistics: May 18 & 19, 2023, in Oxford. Website.
- 2024 Workshop on Recent Advances in Panel and Network Data: June 10 & 11, 2024, in Oxford. Website.
- 2025 Econometrics Workshop : May 29 & 30, 2025, in Oxford. Website.