Graduate Course
SUGGESTED BOOKS
"Data Analysis in High Energy Physics: A Practical Guide to Statistical Methods", Behnke et al. (2013)“
Statistical Data Analysis”, G. Cowan (1998)
"Numerical Recipes", W. Press, S. Teukolsky, W. Vettering & B. Flannery (2007)
“A Guide to the Use of Statistical Methods in the Physical Sciences”, R. J. Barlow (2008)
“Data Analysis: A Bayesian Tutorial” by D. Sivia (1996)
“Measurements and their Uncertainties: A practical guide to modern error analysis” by I. Hughes and T. Hase (2010)
"A Student's Guide to Bayesian Statistics" by Ben Lambert (2018)
“Probability Theory: An Introductory Course” by Ya. G. Sinai (1992)
Example Python Scripts
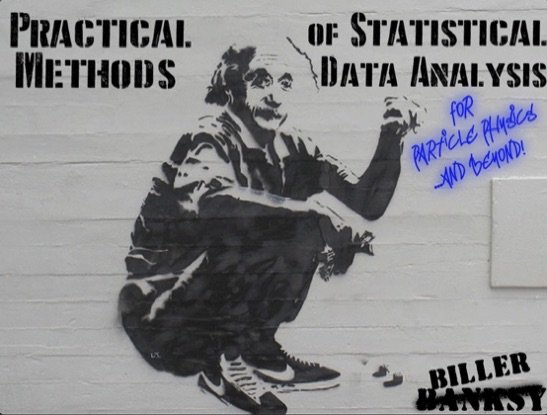